3 questions for...
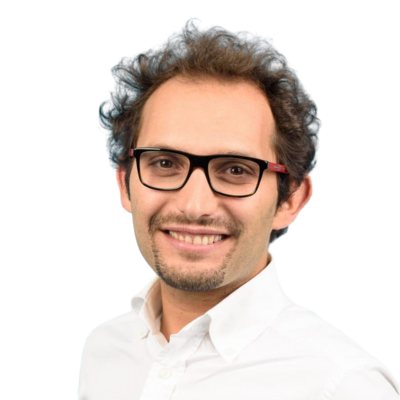
Dr. Marco Fisichella
Mentor
I am an expert in Artificial Intelligence with over 14 years of experience spanning both industry and academia. As the Research Group Leader at L3S, my role encompasses pioneering research in artificial intelligence across fundamental and application-oriented domains. I spearhead the research group at L3S, concentrating on artificial intelligence and intelligent systems. In this capacity, I continue to coordinate and autonomously oversee research projects, ensuring their seamless execution. I remain dedicated to securing new research projects and disseminating our findings through publications at international conferences and specialized journals.
1.
What is needed for research findings to make their way into medical practice?
To translate research findings into medical practice, especially in the context of federated learning (FL), we need scalable and privacy-preserving infrastructures that enable cross-institutional collaboration without compromising data confidentiality. It is crucial that the models are fair, interpretable and generalizable across patient populations. This requires overcoming challenges such as non-IID data, bias in clinical decision making and the interpretability of AI predictions. In addition, adaptation to ethical standards and legal frameworks (e.g. GDPR) is essential for clinical use.
2.
How can sensitive health data be protected without restricting access to high-quality data for medical research?
Sensitive health data can be protected through federated learning, where data remains decentralized and only model updates are shared. To further strengthen data privacy, techniques such as differential privacy, causal modeling and influence function analysis can be integrated. These methods prevent information leakage (e.g., membership inference attacks) while enabling robust privacy-preserving learning from high-quality datasets across institutions. Our project specifically addresses these risks by developing causal privacy preservation methods that go beyond traditional anonymization.
3.
Which factors are most important for patients in building trust in AI-based health technologies?
Trust depends on three key factors: Privacy, fairness and interpretability. Patients need reassurance that their data will not be misused, that the model treats different demographic groups equally and that clinical recommendations can be understood and explained. Our research focuses on interpretable and fair federated models that use causal inference to identify and mitigate bias and attribute predictions to relevant data sources. Transparent communication about these safeguards and a participatory approach to AI development further strengthen patient trust.