Semantic Models
for Medicine
The digital representation of medical knowledge urgently needs improvement. Researchers rely heavily on static, often unstructured text articles to publish their results. Although the existing medical knowledge is partially structured, it is scattered in different vocabularies and scientific databases. These knowledge gaps lead to challenges such as insufficient discoverability, difficult reproducibility and a lack of quality peer review, which jeopardizes the progress of scientific research as a whole.
Recent developments in digital scientific communication have led to new approaches, such as AI-based methods for representing, organizing and retrieving medical knowledge. These approaches use semantic models known as knowledge graphs (KG) to describe research articles, their components (such as research data, software and workflows) and medical knowledge (from medical datasets and vocabularies). One example is the "Open Research Knowledge Graph (ORKG)", developed at the TIB and Leibniz Universität Hannover.
Inductive techniques such as KG embedding and mining models play a crucial role in learning symbolic (e.g., rules) and numerical (e.g., low-dimensional vectors) representations of a KG. These techniques capture explicit and implicit interactions and facilitate various inference tasks, including missing relation prediction, entity alignment, and recommender systems.
Causal models express cause-effect relationships between variables and improve the interpretability of KGs. They support tasks such as counterfactual inference and increase the transferability of predictive models between different populations. Models based on a KG can be more effectively adapted to other KGs with similar causal structures, which extends their utility and generalizability.
This junior research group will explore techniques for orchestrating symbolic, causal reasoning and machine learning to improve the interpretability of AI on medical knowledge from relevant literature, ontologies and databases. The semantic models and hybrid AI methods developed will be available to all CAIMed groups and will be adapted in the CAIMed-ORKG observatory. They are also used for the prediction of interactions and the discovery of patterns to identify RNAs in cardiovascular diseases and pulmonary fibrosis and to develop RNA-targeted therapies.
Team
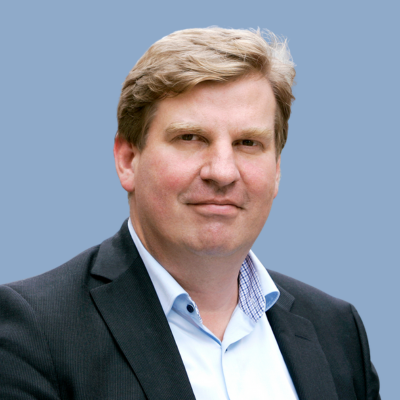
Prof. Dr. Sören Auer, L3S
Mentor
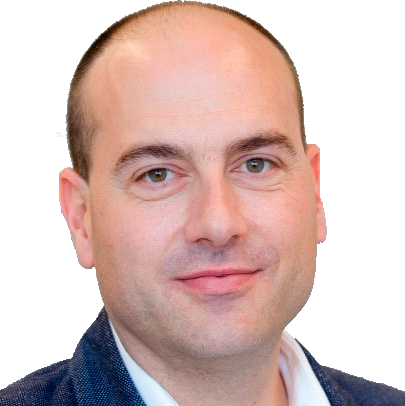
Prof. Dr. Dr. Thomas Thum, MHH
Mentor
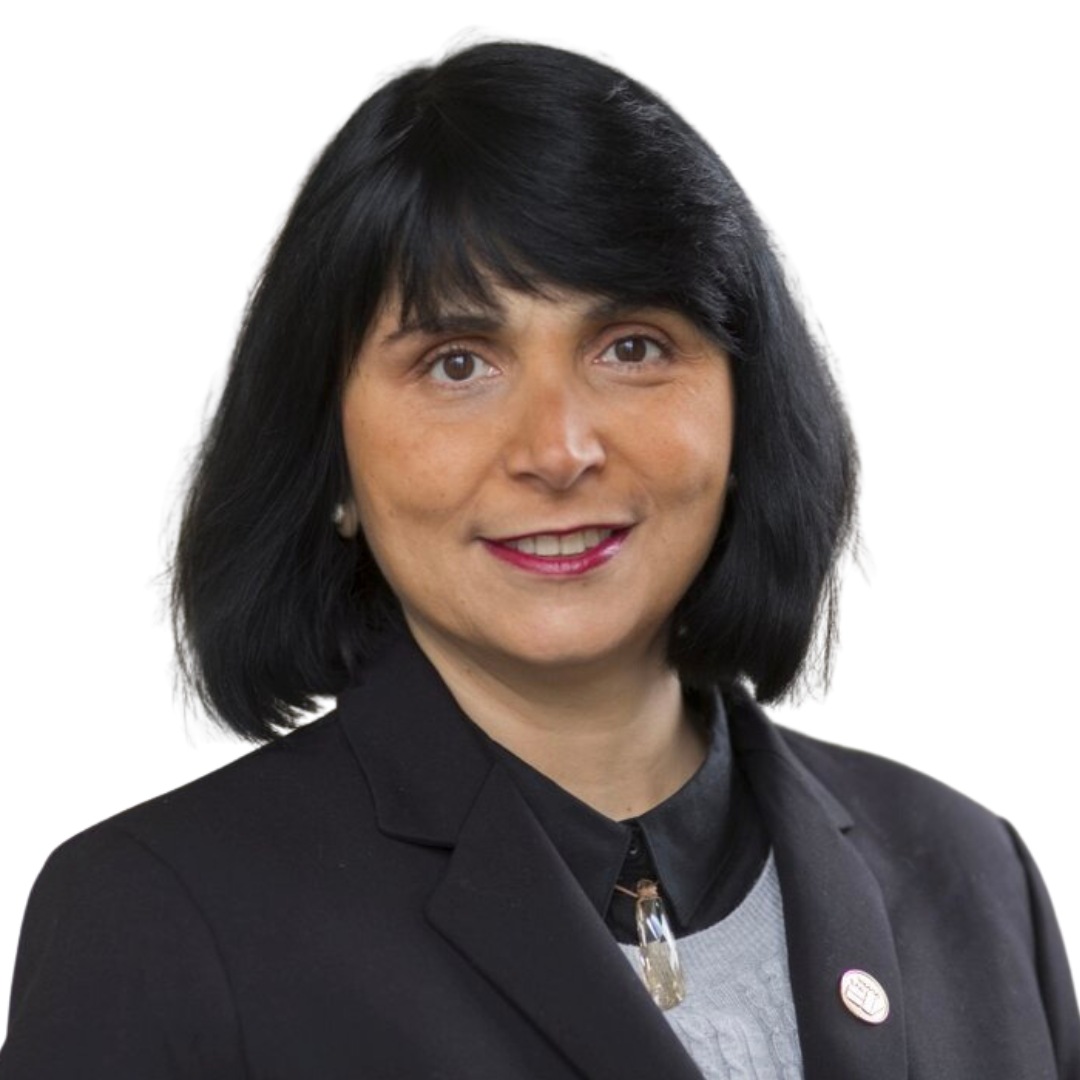
Prof. Dr. Maria-Esther Vidal, L3S
Mentor
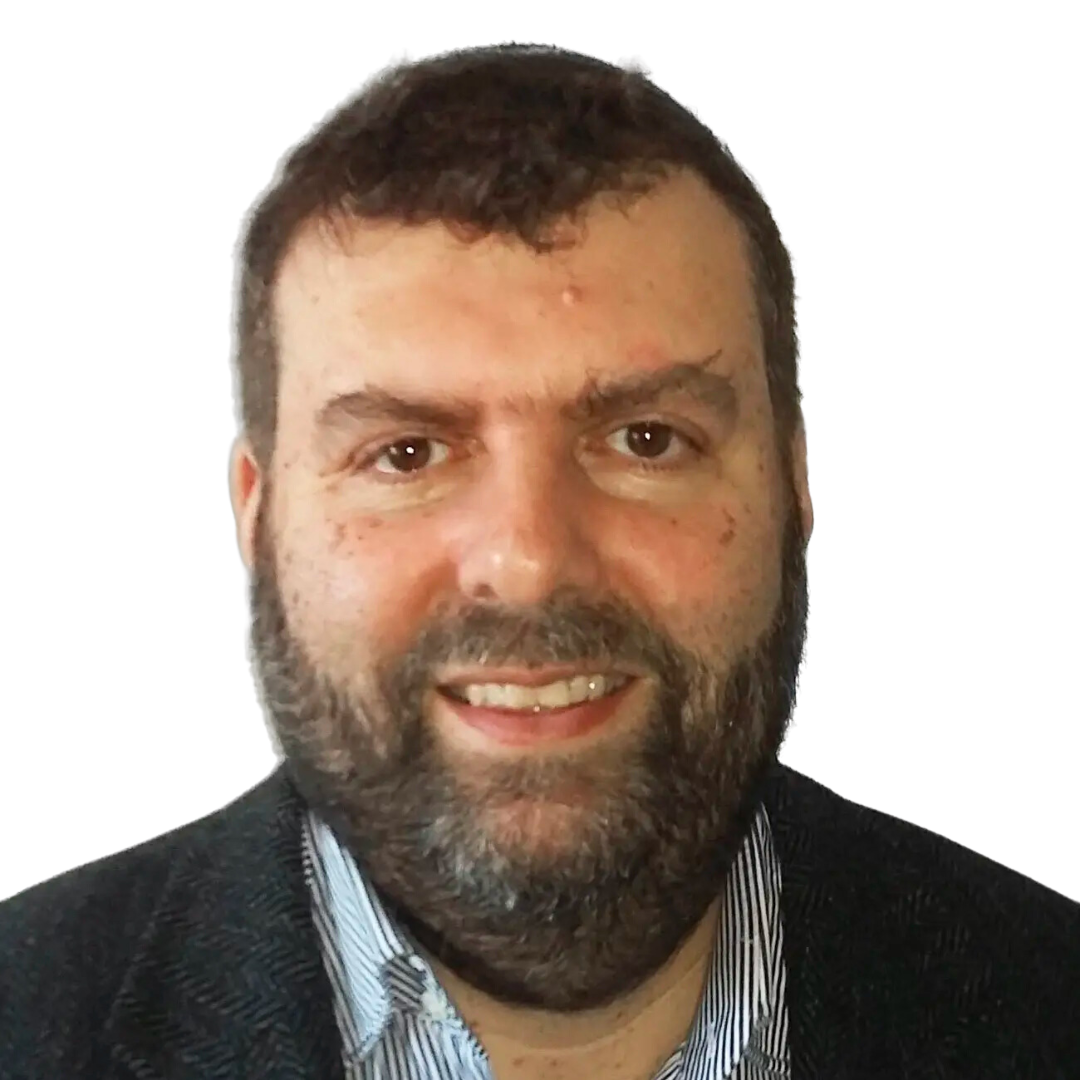
Dr. Daniel Kudenko, L3S
Mentor